The thesis titled “Hand Gestures Recognition using Thermal Images” done by the master student, Daniel Skomedal Breland under the supervision Prof. Linga Reddy Cenkeramaddi has been awarded the best master thesis in ICT for the year 2021.
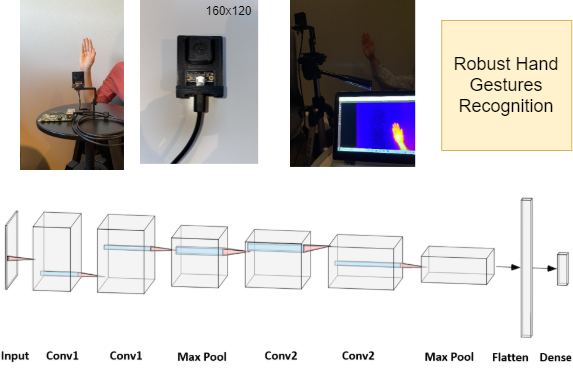
The goal of this project is to develop a robust and reliable hand gesture recognition system using a thermal camera. Hand gestures are an important communication tool for many practical scenarios. It is used in a variety of applications, including medical, entertainment, and industrial settings. The use of human-robot interactions is growing, and there exists several methods. It is possible to gain access to tight and harsh places by using gestures. The majority of gesture recognition is done with RGB cameras, which has the disadvantage of not being able to recognize gestures in low-light situations. Thermal cameras can operate in low-light environments because they are unaffected by external light.